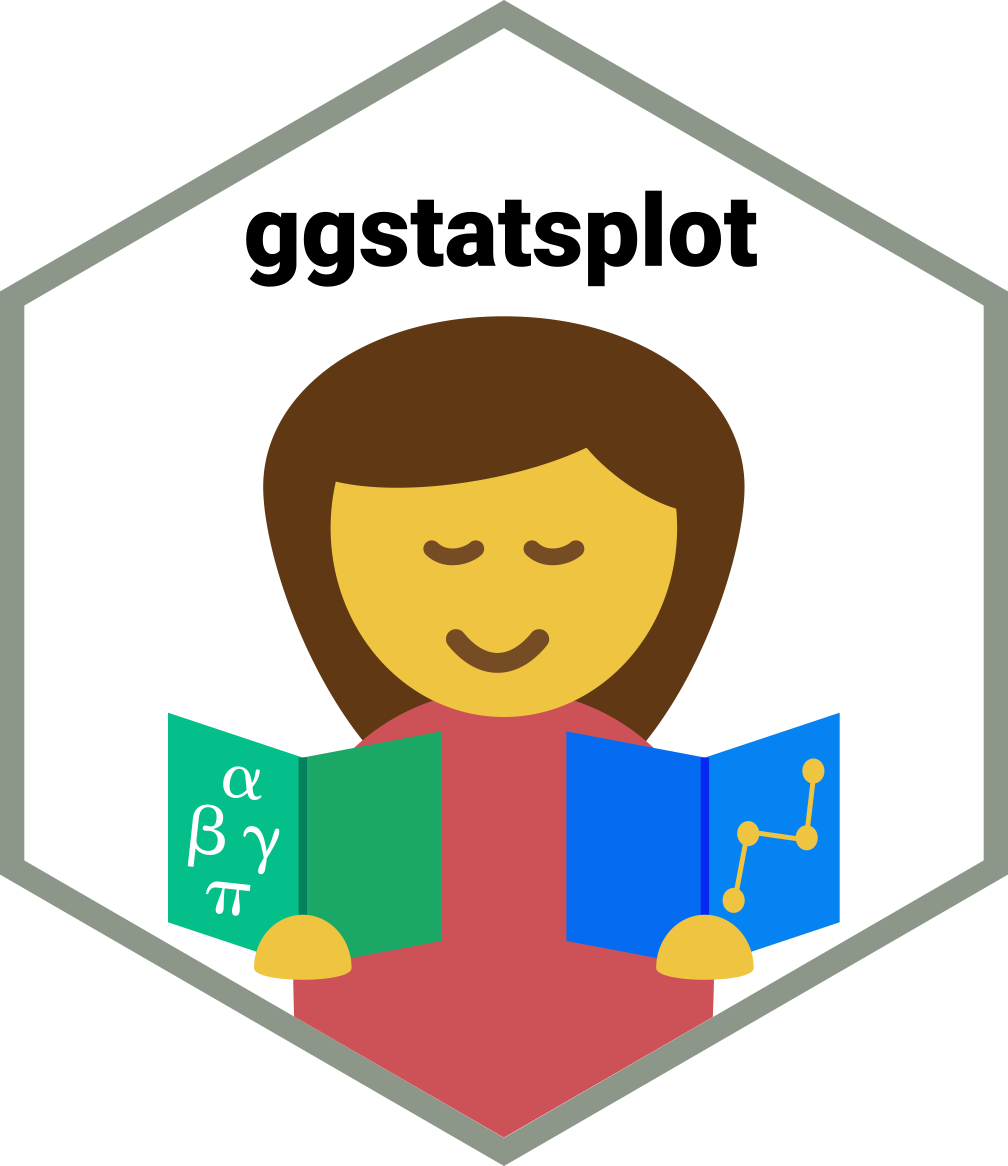
Using 'ggstatsplot' with the 'purrr' package
Source:vignettes/web_only/purrr_examples.Rmd
purrr_examples.Rmd
You can cite this package/vignette as:
To cite package 'ggstatsplot' in publications use:
Patil, I. (2021). Visualizations with statistical details: The
'ggstatsplot' approach. Journal of Open Source Software, 6(61), 3167,
doi:10.21105/joss.03167
A BibTeX entry for LaTeX users is
@Article{,
doi = {10.21105/joss.03167},
url = {https://doi.org/10.21105/joss.03167},
year = {2021},
publisher = {{The Open Journal}},
volume = {6},
number = {61},
pages = {3167},
author = {Indrajeet Patil},
title = {{Visualizations with statistical details: The {'ggstatsplot'} approach}},
journal = {{Journal of Open Source Software}},
}
This is an extremely time-consuming vignette, and so it is not evaluated here. You can still use the code as a reference for writing your own purrr code.
Why use {purrr}
?
Most of the ggstatsplot functions have
grouped_
variants, which are designed to quickly run the
same ggstatsplot function across multiple levels of a
single grouping variable. Although this function is
useful for data exploration, it has two strong weaknesses-
The arguments applied to
grouped_
function call are applied uniformly to all levels of the grouping variable when we might want to customize them for different levels of the grouping variable.Only one grouping variable can be used to repeat the analysis when in reality there can be a combination of grouping variables and the operation needs to be repeated for all resulting combinations.
We will see how to overcome this limitation by combining ggstatsplot with the purrr package.
Note:
While using
purrr::pmap()
, we must input the arguments as strings.You can use ggplot2 themes from extension packages (e.g.
ggthemes
).If you’d like some more background or an introduction to the purrr package, please see this chapter.
Introduction and methodology
For all the examples in this vignette we are going to build
list
s of things that we will pass along to
purrr which will in turn return a list of plots that will
be passed to combine_plots
. As the name implies
combine_plots
merges the individual plots into one bigger
plot with common labeling and aesthetics.
What are these lists
that we are building? The lists
correspond to the parameters in our ggstatsplot function
like ggbetweenstats
. If you look at the help file for
?ggbetweenstats
for example the very first parameter it
wants is the data
file we’ll be using. We can also pass it
different titles
of even ggtheme
themes.
You can pass:
A single character string such as
xlab = "Continent"
or numeric such asnboot = 25
in which case it will be reused/recycled as many times as needed.A vector of values such as
nboot = c(50, 100, 200)
in which case it will be coerced to a list and checked for the right class (in this case integer) and the right quantity of entries in the vector i.e.,nboot = c(50, 100)
will fail if we’re trying to make three plots.A list; either named
data = year_list
or created as you gopalette = list("Dark2", "Set1")
. Any list will be checked for the right class (in this case character) and the right quantity of entries in the list.
ggbetweenstats
Let’s start with ggebtweenstats
. We’ll use the
gapminder
dataset. We’ll make a 3 item list
called year_list
using dplyr::filter
and
split
.
## let's split the data frame and create a list by years of interest
year_list <- gapminder::gapminder %>%
dplyr::filter(year %in% c(1967, 1987, 2007), continent != "Oceania") %>%
split(f = .$year, drop = TRUE)
## checking the length of the list and the names of each element
length(year_list)
names(year_list)
Now that we have the data divided into the three relevant years in a
list we’ll turn to purrr::pmap
to create a list of
ggplot
objects that we’ll make use of stored in
plot_list
. When you look at the documentation for
?pmap
it will accept .l
which is a list of
lists. The length of .l
determines the number of arguments
that .f
will be called with. List names will be used if
present. .f
is the function we want to apply (here,
.f = ggbetweenstats
).
Let’s keep building the list of arguments, .l
. First is
data = year_list
, the x
and y
axes are constant in all three plots so we pass the variable name as a
string x = "continent"
.
## creating a list of plots
plot_list <- purrr::pmap(
.l = list(
data = year_list,
x = "continent",
y = "lifeExp",
xlab = "Continent",
ylab = "Life expectancy",
title = list(
"Year: 1967",
"Year: 1987",
"Year: 2007"
),
type = list("r", "bf", "np"),
pairwise.display = list("s", "ns", "all"),
p.adjust.method = list("hommel", "bonferroni", "BH"),
conf.level = list(0.99, 0.95, 0.90),
digits = list(1, 2, 3),
effsize.type = list(
NULL,
"partial_omega",
"partial_eta"
),
package = list("nord", "ochRe", "awtools"),
palette = list("aurora", "parliament", "bpalette"),
ggtheme = list(
ggthemes::theme_stata(),
ggplot2::theme_classic(),
ggthemes::theme_fivethirtyeight()
)
),
.f = ggbetweenstats
)
The final step is to pass the plot_list
object we just
created to the combine_plots
function. While each of the 3
plots already has labeling information combine_plots
gives
us an opportunity to add additional details to the merged plots and
specify the layout in rows and columns.
## combining all individual plots from the list into a single plot using combine_plots function
combine_plots(
plotlist = plot_list,
annotation.args = list(title = "Changes in life expectancy across continents (1967-2007)"),
plotgrid.args = list(ncol = 1)
)
ggwithinstats
We will be using simulated data from then Attention Network Test
provided in ANT dataset in ez
package.
library(ez)
data("ANT") ## loading data from `ez` package
## let's split the data frame and create a list by years of interest
cue_list <- ANT %>% split(f = .$cue, drop = TRUE)
## checking the length of the list and the names of each element
length(cue_list)
## creating a list of plots by applying the same function for elements of the list
plot_list <- purrr::pmap(
.l = list(
data = cue_list,
x = "flank",
y = "rt",
xlab = "Flank",
ylab = "Response time",
title = list(
"Cue: None",
"Cue: Center",
"Cue: Double",
"Cue: Spatial"
),
type = list("p", "r", "bf", "np"),
pairwise.display = list("ns", "s", "ns", "all"),
p.adjust.method = list("fdr", "hommel", "bonferroni", "BH"),
conf.level = list(0.99, 0.99, 0.95, 0.90),
digits = list(3, 2, 2, 3),
effsize.type = list(
"omega",
"eta",
"partial_omega",
"partial_eta"
),
package = list("ggsci", "palettetown", "palettetown", "wesanderson"),
palette = list("lanonc_lancet", "venomoth", "blastoise", "GrandBudapest1"),
ggtheme = list(
ggplot2::theme_linedraw(),
hrbrthemes::theme_ft_rc(),
ggthemes::theme_solarized(),
ggthemes::theme_gdocs()
)
),
.f = ggwithinstats
)
## combining all individual plots from the list into a single plot using combine_plots function
combine_plots(
plotlist = plot_list,
annotation.args = list(title = "Response times across flank conditions for each type of cue"),
plotgrid.args = list(ncol = 1)
)
ggscatterstats
For the next example lets use the same methodology on different data
and using ggscatterstats
to produce scatterplots combined
with marginal histograms/boxplots/density plots with statistical details
added as a subtitle. For data we’ll use movies_long
which
is from IMDB and part of the ggstatsplot package. Since
it’s a large dataset with some relatively small categories like
NC-17 we’ll sample only one quarter of the data and
completely drop NC-17 using dplyr
.
This time we’ll put all the code in one block-
mpaa_list <- movies_long %>%
dplyr::filter(mpaa != "NC-17") %>%
dplyr::sample_frac(size = 0.25) %>%
split(f = .$mpaa, drop = TRUE)
## creating a list of plots
plot_list <- purrr::pmap(
.l = list(
data = mpaa_list,
x = "budget",
y = "rating",
xlab = "Budget (in millions of US dollars)",
ylab = "Rating on IMDB",
title = list(
"MPAA Rating: PG",
"MPAA Rating: PG-13",
"MPAA Rating: R"
),
label.var = list("title"),
## note that you need to quote the expressions
label.expression = list(
quote(rating > 7.5 & budget < 100),
quote(rating > 8 & budget < 50),
quote(rating > 8 & budget < 10)
),
type = list("r", "np", "bf"),
xfill = list("#009E73", "#999999", "#0072B2"),
yfill = list("#CC79A7", "#F0E442", "#D55E00"),
ggtheme = list(
ggthemes::theme_tufte(),
ggplot2::theme_classic(),
ggplot2::theme_light()
)
),
.f = ggscatterstats
)
## combining all individual plots from the list into a single plot using combine_plots function
combine_plots(
plotlist = plot_list,
annotation.args = list(
title = "Relationship between movie budget and IMDB rating",
caption = "Source: www.imdb.com"
),
plotgrid.args = list(ncol = 1)
)
The remainder of the examples vary in content but follow the exact same methodology as the earlier examples.
ggcorrmat
## splitting the data frame by cut and creating a list
## let's leave out "fair" cut
## also, to make this fast, let's only use 5% of the sample
cut_list <- ggplot2::diamonds %>%
dplyr::sample_frac(size = 0.05) %>%
dplyr::filter(cut != "Fair") %>%
split(f = .$cut, drop = TRUE)
## checking the length and names of each element
length(cut_list)
names(cut_list)
## running function on every element of this list note that if you want the same
## value for a given argument across all elements of the list, you need to
## specify it just once
plot_list <- purrr::pmap(
.l = list(
data = cut_list,
cor.vars = list(c("carat", "depth", "table", "price")),
type = list("pearson", "np", "robust", "bf"),
partial = list(TRUE, FALSE, TRUE, FALSE),
title = list("Cut: Good", "Cut: Very Good", "Cut: Premium", "Cut: Ideal"),
p.adjust.method = list("hommel", "fdr", "BY", "hochberg"),
lab.size = 3.5,
colors = list(
c("#56B4E9", "white", "#999999"),
c("#CC79A7", "white", "#F0E442"),
c("#56B4E9", "white", "#D55E00"),
c("#999999", "white", "#0072B2")
),
ggtheme = list(
ggplot2::theme_linedraw(),
ggplot2::theme_classic(),
ggthemes::theme_fivethirtyeight(),
ggthemes::theme_tufte()
)
),
.f = ggcorrmat
)
## combining all individual plots from the list into a single plot using
## `combine_plots` function
combine_plots(
plotlist = plot_list,
guides = "keep",
annotation.args = list(
title = "Relationship between diamond attributes and price across cut",
caption = "Dataset: Diamonds from ggplot2 package"
),
plotgrid.args = list(nrow = 2)
)
gghistostats
## let's split the data frame and create a list by continent
## let's leave out Oceania because it has just two data points
continent_list <-
gapminder::gapminder %>%
dplyr::filter(year == 2007, continent != "Oceania") %>%
split(f = .$continent, drop = TRUE)
## checking the length and names of each element
length(continent_list)
names(continent_list)
## running function on every element of this list note that if you want the same
## value for a given argument across all elements of the list, you need to
## specify it just once
plot_list <-
purrr::pmap(
.l = list(
data = continent_list,
x = "lifeExp",
xlab = "Life expectancy",
test.value = list(35.6, 58.4, 41.6, 64.7),
type = list("p", "np", "r", "bf"),
bf.message = list(TRUE, FALSE, FALSE, FALSE),
title = list(
"Continent: Africa",
"Continent: Americas",
"Continent: Asia",
"Continent: Europe"
),
effsize.type = list("d", "d", "g", "g"),
ggtheme = list(
ggplot2::theme_classic(),
hrbrthemes::theme_ipsum_tw(),
ggplot2::theme_minimal(),
hrbrthemes::theme_modern_rc()
)
),
.f = gghistostats
)
## combining all individual plots from the list into a single plot using combine_plots function
combine_plots(
plotlist = plot_list,
annotation.args = list(
title = "Improvement in life expectancy worldwide since 1950",
caption = "Note: black line - 1950; blue line - 2007"
),
plotgrid.args = list(nrow = 4)
)
ggdotplotstats
library(ggthemes)
library(hrbrthemes)
## let's split the data frame and create a list by continent
## let's leave out Oceania because it has just two data points
continent_list <-
gapminder::gapminder %>%
dplyr::filter(continent != "Oceania") %>%
split(f = .$continent, drop = TRUE)
## checking the length and names of each element
length(continent_list)
names(continent_list)
## running function on every element of this list note that if you want the same
## value for a given argument across all elements of the list, you need to
## specify it just once
plot_list <-
purrr::pmap(
.l = list(
data = continent_list,
x = "gdpPercap",
y = "year",
xlab = "GDP per capita (US$, inflation-adjusted)",
test.value = list(2500, 9000, 9500, 10000),
type = list("p", "np", "r", "bf"),
title = list(
"Continent: Africa",
"Continent: Americas",
"Continent: Asia",
"Continent: Europe"
),
effsize.type = list("d", "d", "g", "g"),
centrality.line.args = list(
list(color = "red"),
list(color = "#0072B2"),
list(color = "#D55E00"),
list(color = "#CC79A7")
),
ggtheme = list(
ggplot2::theme_minimal(base_family = "serif"),
ggthemes::theme_tufte(),
hrbrthemes::theme_ipsum_rc(axis_title_size = 10),
ggthemes::theme_hc(bgcolor = "darkunica")
)
),
.f = ggdotplotstats
)
## combining all individual plots from the list into a single plot using combine_plots function
combine_plots(
plotlist = plot_list,
annotation.args = list(title = "Improvement in GDP per capita from 1952-2007"),
plotgrid.args = list(nrow = 4),
guides = "keep"
)
ggpiestats
## let's split the data frame and create a list by passenger class
class_list <- Titanic_full %>% split(f = .$Class, drop = TRUE)
## checking the length and names of each element
length(class_list)
names(class_list)
## running function on every element of this list note that if you want the same
## value for a given argument across all elements of the list, you need to
## specify it just once
plot_list <-
purrr::pmap(
.l = list(
data = class_list,
x = "Survived",
y = "Sex",
label = list("both", "count", "percentage", "both"),
title = list(
"Passenger class: 1st",
"Passenger class: 2nd",
"Passenger class: 3rd",
"Passenger class: Crew"
),
caption = list(
"Total: 319, Died: 120, Survived: 199, % Survived: 62%",
"Total: 272, Died: 155, Survived: 117, % Survived: 43%",
"Total: 709, Died: 537, Survived: 172, % Survived: 25%",
"Data not available for crew passengers"
),
package = list("RColorBrewer", "ghibli", "palettetown", "yarrr"),
palette = list("Accent", "MarnieMedium1", "pikachu", "nemo"),
ggtheme = list(
ggplot2::theme_grey(),
ggplot2::theme_bw(),
ggthemes::theme_tufte(),
ggthemes::theme_economist()
),
proportion.test = list(TRUE, FALSE, TRUE, FALSE),
type = list("p", "p", "bf", "p")
),
.f = ggpiestats
)
## combining all individual plots from the list into a single plot using combine_plots function
combine_plots(
plotlist = plot_list,
annotation.args = list(title = "Survival in Titanic disaster by gender for all passenger classes"),
plotgrid.args = list(ncol = 1),
guides = "keep"
)
ggbarstats
## let's split the data frame and create a list by passenger class
class_list <- Titanic_full %>% split(f = .$Class, drop = TRUE)
## checking the length and names of each element
length(class_list)
names(class_list)
## running function on every element of this list note that if you want the same
## value for a given argument across all elements of the list, you need to
## specify it just once
plot_list <-
purrr::pmap(
.l = list(
data = class_list,
x = "Survived",
y = "Sex",
type = "bayes",
label = list("both", "count", "percentage", "both"),
title = list(
"Passenger class: 1st",
"Passenger class: 2nd",
"Passenger class: 3rd",
"Passenger class: Crew"
),
caption = list(
"Total: 319, Died: 120, Survived: 199, % Survived: 62%",
"Total: 272, Died: 155, Survived: 117, % Survived: 43%",
"Total: 709, Died: 537, Survived: 172, % Survived: 25%",
"Data not available for crew passengers"
),
package = list("RColorBrewer", "ghibli", "palettetown", "yarrr"),
palette = list("Accent", "MarnieMedium1", "pikachu", "nemo"),
ggtheme = list(
ggplot2::theme_grey(),
ggplot2::theme_bw(),
ggthemes::theme_tufte(),
ggthemes::theme_economist()
)
),
.f = ggbarstats
)
## combining all individual plots from the list into a single plot using combine_plots function
combine_plots(
plotlist = plot_list,
annotation.args = list(
title = "Survival in Titanic disaster by gender for all passenger classes",
caption = "Asterisks denote results from proportion tests: \n***: p < 0.001, ns: non-significant"
),
plotgrid.args = list(ncol = 1),
guides = "keep"
)
grouped_
variants
Note that although all the above examples were written with the
non-grouped variants of functions, the same rule holds true for the
grouped_
variants of all the above functions.
For example, if we want to use the grouped_gghistostats
across three different datasets, you can use purrr::pmap()
function. For the sake of brevity, the plots are not displayed here, but
you can run the following code and check the individual
grouped_
plots (e.g., plotlist[[1]]
).
## create a list of plots
plotlist <- purrr::pmap(
.l = list(
data = list(mtcars, iris, ToothGrowth),
x = alist(wt, Sepal.Length, len),
results.subtitle = list(FALSE),
grouping.var = alist(am, Species, supp)
),
.f = grouped_gghistostats
)
## given that we had three different datasets, we expect a list of length 3
## (each of which contains a `grouped_` plot)
length(plotlist)
Repeating function execution across multiple columns in a data frame
library(patchwork)
## running the same analysis on two different columns (creates a list of plots)
plotlist <- purrr::pmap(
.l = list(
data = list(movies_long),
x = "mpaa",
y = list("rating", "length"),
title = list("IMDB score by MPAA rating", "Movie length by MPAA rating")
),
.f = ggbetweenstats
)
## combine plots using `patchwork`
plotlist[[1]] + plotlist[[2]]
Suggestions
If you find any bugs or have any suggestions/remarks, please file an
issue on GitHub
: https://github.com/IndrajeetPatil/ggstatsplot/issues